Increase Revenue by 21% by Optimizing Marketing Strategies with Predictive Customer Journeys
- Linda Orr
- Oct 24, 2024
- 3 min read
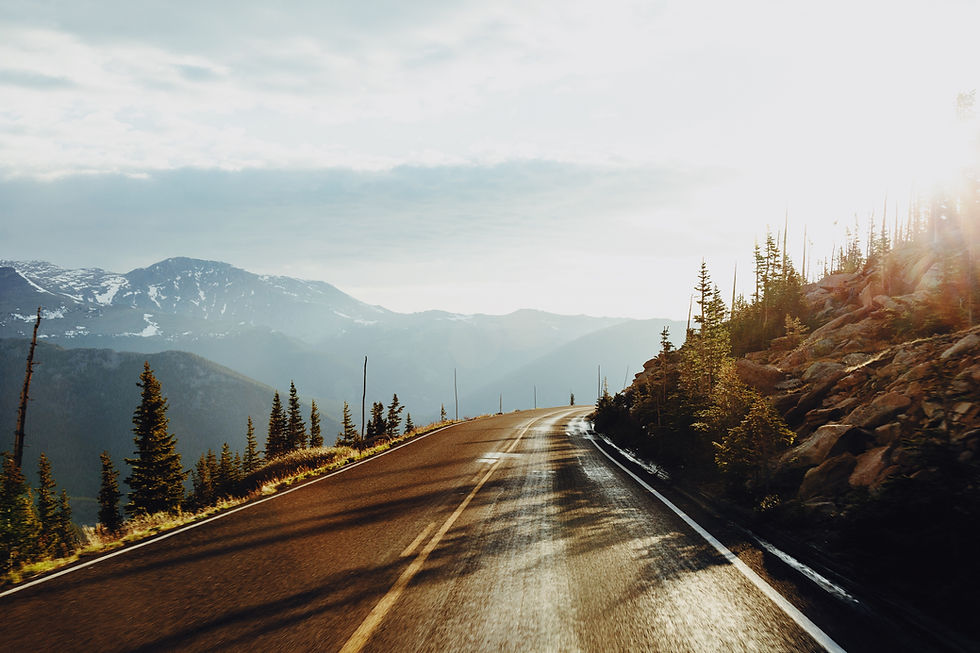
In the competitive realm of digital marketing, leveraging predictive customer journey modeling has proven to be a game-changer for businesses aiming to enhance their marketing strategies and boost financial outcomes.
Revenue Increase: Companies using predictive analytics have witnessed a revenue increase by up to 21% compared to those who do not, underscoring the significant impact of leveraging advanced data analysis. (Source: Forbes)
Margin Improvement: Businesses that implement machine learning for personalization in their marketing efforts see, on average, a 15% improvement in their margins. (Source: McKinsey)
Customer Retention: According to a study by Gartner, organizations that have fully invested in all types of online personalization will outsell companies that have not by 30%.
ROI from Marketing Investments: Forrester reports that insight-driven businesses are growing at an average of more than 30% annually and are on track to earn $1.8 trillion by 2021.
These statistics clearly demonstrate how predictive modeling can significantly enhance the efficiency of marketing campaigns, driving substantial improvements in both top-line growth and bottom-line profitability. Let’s explore how to implement predictive customer journeys to achieve these benefits.
How to Implement Predictive Customer Journey Modeling
Gather Historical Data: Collecting a comprehensive set of data is essential for developing predictive models that accurately forecast customer behaviors. Start by integrating data from a variety of sources that track customer interactions across different touchpoints. For instance, website analytics can provide insights into user behavior such as which pages they visit most frequently and how long they stay on each page. CRM systems can offer valuable data on purchase history, showing patterns in buying behavior, while interactions from social media platforms can reveal preferences and sentiments towards certain products or campaigns. Additionally, data from offline interactions should not be overlooked as they contribute to a complete customer profile.
Choose the Right Machine Learning Model: Selecting an appropriate machine learning model is crucial for predicting customer behavior with high accuracy. Regression models can be applied to forecast quantitative outcomes like spending amounts, while clustering algorithms are perfect for identifying groups within your data, helping to segment customers by behavior or demographics. Classification algorithms are useful for predicting categorical outcomes, such as classifying customers by their likelihood to purchase again or churn. Tools like Python's Scikit-Learn offer a user-friendly approach to implementing these models, providing a range of options that can be tailored to your specific data characteristics. Statistical programs are more user friendly now than ever before. If you are hiring a statistician or modelers, make sure they understand marketing strategy as well. You can make data say anything you want it to say, so models must be set up with a solid strategic mindset.
Analyze and Model Data: Once you have selected your model, the next step is to use it to analyze historical data and identify patterns that predict future behavior. This involves looking for trends and insights that inform you about your customers’ pathways through the sales funnel and potential drop-off points. This deep dive into the data helps you understand not just what actions your customers are likely to take next, but also why they might take them, allowing for more targeted and strategic interventions. Repeat these analyzes. This is not a set it and forget it system.
Personalize the Customer Experience: Use the insights gained from your predictive analysis to tailor the customer journey at every step. For example, if your model indicates that a certain segment of customers tends to research a specific product type extensively before making a purchase, you can automate personalized communications such as emails or targeted ads that provide useful information or timely incentives related to those products. This level of personalization makes customers feel understood and valued, which can significantly enhance their experience and increase their loyalty to your brand.
Continuously Refine Your Model: Predictive models require continuous refinement and updates to maintain their accuracy over time. Regularly integrate new customer data into the model to keep it current and reflective of the latest customer behaviors and trends. Establish a feedback loop that incorporates customer reactions and real-time feedback into the model, allowing for dynamic adjustments. It's also important to regularly assess the performance of your model by monitoring its predictions against actual customer behaviors and making necessary adjustments. This ongoing process of refinement ensures that your predictive model remains a reliable and effective tool for understanding and anticipating customer needs.
Implementing predictive customer journeys allows businesses to stay a step ahead of customer expectations, offering personalized experiences that lead to increased satisfaction and loyalty. By anticipating customer needs and customizing interactions, companies can significantly enhance their marketing effectiveness and drive sustainable growth.
Ready to transform your customer journey with predictive analytics? Contact Orr Consulting to integrate advanced machine learning models into your marketing strategy and unlock new levels of customer engagement and satisfaction.
Comments